This article originally appeared on Law360 Pulse on February 21, 2024. View the original article here.
In a 2023 survey,[1] litigation support company U.S. Legal Support asked lawyers and legal professionals what legal tech trends emerged during the year and how they expect their use of technology to change in 2024.
Predictably, the survey noted significant growth in the use of generative artificial intelligence tools within the legal industry, predicting further expansion this year. Legal organizations are being challenged by the pace of change, struggling to work out the right investment strategy.
The answer to this challenge is to get back to basic principles. The model for optimal legal department efficiency is not difficult to articulate. It is based on an understanding of where workloads belong, according to the basic functions and priorities of a corporate legal team.
The model does not depend on generative AI, nor indeed on any particular technology or tool. Generative AI offers the exciting prospect of helping to shift some types of work from one part of the model to another — but without changing the basic construction of the model. Let’s call this model, which categorizes workloads, the Matrix.[2]
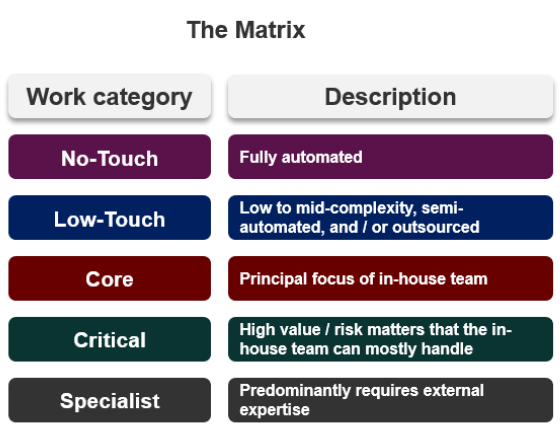
No-Touch
No-touch workloads are fully automated: They do not require any handling by legal professionals.
The classic example is purchase order processing on standard terms. A less dated example would be the chatbot. Properly designed, an AI-powered chatbot can automate a huge range of activities that traditionally required a live interface between business client and legal professional.
Scenarios include the selection of a standard legal document for a particular transaction, and user Q&A resulting in the delivery to a client of a FAQs sheet on a given legal topic. The power of the generative AI tool is to make the client engagement experience natural and intuitive.
Low-Touch
According to the Matrix, low-touch workloads are of low to mid complexity and either semi-automated or outsourced. Outsourcing means shifting the workload as efficiently as possible away from the corporate legal department.
Traditionally, the work might have been outsourced to a law firm, but because law firms offer specialist expertise on tap, that would be the most expensive form of legal support. Low-touch work should be in the least expensive category.
Historically, this is the category where we have seen the biggest concentration of corporate inefficiency. Until the emergence of alternative legal service providers, or ALSPs, low-touch work was either outsourced to a law firm, sucked up by the in-house team or staffed by the department, hiring more employees or using interim contractors.
ALSPs emerged to provide a more cost-efficient model for this category of workload, offering process simplification and standardization coupled with tight management of a frequently offshored team of legal professionals. Depending on the type of work involved, technology has become an increasing feature of the ALSP value proposition — think e-discovery, for example.
I’m going to say something controversial: Generative AI is introducing confusion into the marketplace. Legal departments are reading and hearing that the technology can drive radical levels of automation, scale and speed into workloads that previously demanded in-house or external resources.
The idea is that the technology can take over work that people were handling, so the race is on to invest in use-case development to operationalize this capability. The point of confusion is that many corporate legal teams believe that they should be licensing the generative AI tools and working on the design and build of the solutions. If they are doing that for low-touch work, they are making a mistake.
Building a generative AI solution for low-touch work is not a one-time event. The tool will require ongoing quality control, for starters.
But there is another big issue to consider. The industry is just at the beginning of the generative AI revolution. There are so many tech vendors to choose from, and indeed the optimal design features of the tools are still taking shape — for example, with selections being made about the best large language model menu options that should be used to provide the foundations of the solution.
So here is the point: Law departments building their own solutions are being asked to place a bet on the right technology, and then to make the necessary resourcing investments for the ongoing process management and continuous improvement. These commitments might make sense for high-value workloads, but not for low-touch matters. For low-touch workloads, in-house teams should rely heavily on their technology and service partners to bring generative AI to the table and ensure that the work is managed to high standards, low prices, maximum efficiency and continuing improvement.
Why? Because then the legal department can start focusing on fully automated solutions — no-touch work — and on how to innovate around its core activity.
This is the paradox. By being drawn into investments of time, money and energy focused on building generative AI use cases for low-touch work, legal departments are actually diverting attention away from the areas where they should really be innovating.
Core
The Matrix describes the core workload as being the principal focus of the in-house team. There are two perspectives to consider: the company itself, and the lawyers who make up the department.
From the company’s point of view, a legal department is worth having if the business requires similar types of legal support on a sufficiently frequent basis to warrant employing its own lawyers rather than instructing external counsel.
The advantages of the in-house team go beyond simply saving money, though, because the employee can build relationships internally and develop institutional and industrial knowledge that may be hard for the external lawyer to match. And from the perspective of the lawyer, a major attraction of an in-house role is precisely that ability to build deep connections with the business client and establish levels of commercial influence that few external advisers could aspire to.
So we can develop our definition of core workload along the following lines. The type of work should:
- Be valuable to the business client and proportional to the cost of the adviser, e.g. preferably time spent advising on a sensitive customer dispute rather than chasing a master service agreement signature;
- Not readily be capable of automation, because if it is, it should be no-touch;
- Not be repeatable, low- to mid-complexity activity that can be outsourced cost-effectively; and
- Come up sufficiently frequently that it makes sense to employ the adviser rather than instruct external counsel or a temporary contractor.
Returning to the third criterion, why would cost-effective outsourcing be preferable to allocating the work to the in-house team? Because:
- This is repeatable, low- to mid-complexity work, meaning that the activity will be less appealing in the long run to the in-housers who want to build sophisticated, commercial advisory relationships with their clients.
- This type of workload often fluctuates in volume over the year. A good service provider will offer a delivery framework that has affordable scalability built in. The alternative is to intermittently weigh down the in-house team with work that is both burdensome and uninspiring, or resort to temporary and more expensive overspill solutions.
- The service provider can be given responsibility for innovation and technology enablement for the workload, including choosing and licensing the right tools and ensuring that continuous improvement is part of the package.
So the challenge of building generative AI tools into a support package for low or mid-complexity work should fall to the solution providers, leaving the in-house team free to focus on generative AI, other technologies and innovations to drive efficiency, and service enhancement in core workloads.
The list of potential use cases for generative AI in core workloads is vast, if not unlimited.
Imagine that your company has a small in-house legal team, and a services business that is relentlessly invited to engage with potential partners under memoranda of understanding, or MOUs. No matter how often the same broad topics come up in these opportunities, every engagement is different and each MOU has its own idiosyncratic demands in terms of format, priorities, style of response, etc.
Right now, producing these MOUs is core business for the legal team, and specifically for you as the MOU legal point of contact for your clients. Imagine being able to load the last 24 months worth of MOUs into a repository, and then do an analysis of those agreements with a view to producing a standard version with playbook alternatives.
Imagine further a chatbot that allows the client to generate their own first draft of an MOU based on the playbook parameters, with that draft then coming to you for quality assurance and fact validation.
This is an example of how generative AI can help move workloads from one Matrix category to another. Here, the work would go from the Core category to the Low-Touch category, becoming semi-automated in the process. If you have an ALSP on board for low-touch work, that activity could then be added to the outsourced tasks for maximum efficiency, freeing you up to move on from your MOU role and really demonstrate commercial value.
Critical
Critical workloads are characterized by the highest value and/or risk for the corporation, like big deals, significant disputes and major regulatory change programs.
But this is still work that the in-house team can mostly handle on their own. A merger or acquisition will typically fall into the Critical category, but different legal departments have varying levels of resourcing for such work. A major multinational with an acquisitive history will very likely maintain its own team of M&A lawyers. Not so a fast-growing startup, who might need to engage specialist outside counsel.
Staying with the M&A example, it is clear that advising on deal structure will usually require a degree of legal expertise, commercial judgment, etc., that is anything but low-touch. But what about due diligence?
There are aspects of the due diligence exercise that can be highly standardized and semi-automated with machine learning and/or generative AI tools. Process discipline, quality control and accuracy are key requirements — activities that are often managed to high standards by ALSPs who specialize in what we could call “repeatable work at scale.” And now we are seeing off-the-shelf generative AI tools with immediate applicability to M&A activity.
Critical workloads can mostly be handled by the in-house team, taking into account that team’s size and range of expertise. Therein lies the difference with the final Matrix category: Specialist.
Specialist
The Specialist category covers workloads that predominantly demand external expertise. The circumstances for such a requirement will vary.
For example, the in-house HR lawyer might be on maternity leave, so that a temporary specialist needs to be contracted. Or the senior intellectual property lead could be working 12 hours a day on a big trademark dispute, such that external counsel needs to be brought in for a patent-heavy tech transaction.
The in-house community will rely on their external advisers to drive the development of generative AI use cases in the Specialist category, because of the expertise involved. But these use cases can only be built using the right datasets, and it is often the clients who are the proprietors of those datasets.
Getting data generative-AI-ready is itself a resource-intensive activity, so even where the law firm and tech provider are proposing to take the lead role in building use cases for specialist work, in many instances the clients will need to dedicate their own time and people to the development project.
This is the challenge with generative AI in the corporate legal sphere. The technology promises remarkable productivity gains,[3] but only with the appropriate investments in time, resources and management focus.
Legal departments will have to make smart choices about where to make those investments, and the present blizzard of generative AI reports, product announcements and opinion pieces can make it hard to decide.
[1] https://www.jdsupra.com/legalnews/survey-results-predict-top-legal-9393251/
[2] Because this is “a grid-like arrangement of elements” and not just because everybody loves Keanu Reeves.
[3] Last year Gartner’s 2026 Strategic Planning Assumptions stated that 25% of first-draft contracts and other corporate legal written documents will be generated “entirely by AI tools.”